Research Highlight How to encourage managers to adopt AI for decision-making
With the meteoritic rise of AI, many questions arise about the use of algorithms to enhance decision-making and improve organizational performance. Yet a sizable number of managers are reluctant to adopt algorithmic decision-making in the first place. Here are some suggestions to identify and overcome individual barriers to algorithmic decision-making.
In just a few years, the lightning advances in artificial intelligence (AI), the use of big data and the increase in processing capacity have revolutionized most sectors, with organizations increasingly relying on algorithms for decisions they now define as ‘smart’ (and while they're at it, often rebranding processes, or the entire business as such).
Already as far back as 2019, a survey of over 2,500 executives conducted by MIT Sloan Management Review and Boston Consulting Group found that most companies had begun to invest in and use AI capabilities. “Algorithmic decision-making is already being deployed in many industries, including banking, energy, science, psychological counselling, health care, public administration, transportation, legal counselling, insurance, retail and so forth,” details a recent article based on Anniek Brink’s Master’s thesis. Applications range from facial recognition to translation, from medical diagnosis to anticipating crime and from fighting fraud to influencing what people buy, read and vote. The much-debated launch of ChatGPT in November 2022 “has brought the potential of AI to support and potentially even replace human decision-making to a large audience.”
Not all of the audience has jumped as enthusiastically on the bandwagon, though. A not insignificant proportion of managers are digging their heels in. Consciously or unconsciously, they prefer to follow their instincts (or other people's) rather than trust algorithmic decisions – despite algorithms frequently outperforming human decision-makers, as the authors point out. This phenomenon is called ‘algorithm aversion’.
The factors behind ‘algorithm aversion’
Why do individuals distrust AI? And more to the point, how to overcome this distrust, which may harm organizational performance when managers doggedly neglect AI-based recommendations?
The authors found no less than 18 different factors behind this reluctance, identified in previous studies, which they clustered into four categories: familiarity, psychology, demography and personality.
- Familiarity
In the interviews the academics conducted, familiarity with AI technology appeared as the main driver of AI adoption. On the emotional level, some individuals may have a negative perception of AI because they associate it with dangers depicted in science-fiction films like Steven Spielberg's Minority Report. On a more pragmatic plane, they may simply not understand AI systems, or perceive them as unreliable, especially if they have had a bad experience in the past – not that humans don't make mistakes either, as the authors point out, but “it bothers managers much more when the output of an algorithm is incorrect.” - Psychology
Managers may be hindered by a lack of emotional connection with AI systems or by a “moral obligation to pursue one's judgement,” especially if they place overly high expectations in AI systems. - Demographics
Resistance is stronger among the older generation, more because of confidence in years of experience than because of age itself, and among those with a lower educational level. - Personality
The sense of control over one's decisions, self-esteem and self-efficacy (confidence in the ability to reach one's goal), as well as extraversion and risk appetite are all predictive of AI adoption.
How to foster AI adoption
The authors propose the following strategies to address these barriers:
- Emphasize the benefits through tailored information and training
Scepticism may be combatted by providing concrete examples of how the system has improved decision-making in other contexts and highlighting the benefits the algorithm will bring to the specific job being considered: cost savings, 24/7 availability, taking over repetitive jobs, etc. But a one-size-fits-all training won't do; instead, tailor it to the level of education and confidence of your managers. - Explain how the algorithms work and be transparent on the shortcomings
Algorithms are often compared to black boxes, with no one really knowing what goes on inside. While not all managers can understand the actual code, it is important that they gain a notion of how algorithms operate. The researchers recommend first implementing simple models before moving on to more complex ones and especially acknowledging biases or possible errors. - Strike a good balance between automated and human-made decisions
The more users feel they are losing control, the more reluctant they are. The problem can be solved by allowing users to retain some level of control over the decision-making process, for example with the ability to adjust the weighting on different criteria or to override the eventual decision. It is also advisable to start using algorithms at the operational level, for monotonous tasks, and then move to complex, less structured decisions. The authors suggest embodying the balance between automated and human-made decisions in a policy that would specify which decisions can be automated, which should be supported by AI (and to which degree) or reserved for humans. - Involve users in the design process
While the idea is not to get users to fall in love with their virtual assistants like Spike Jonze's character in the film Her, involving end-users in the design process can help to develop an emotional connection with algorithmic decision-making. It can also change their belief about algorithm performance. A key feature of agile and design thinking methodologies for software and product development, user feedback can help developers identify and address issues.
Even though algorithmic decision-making is a clear source of performance improvement through the automation of decisions, it should not be concluded that humans are out of the loop. Humans decide when decisions should (and should not) be made by algorithms.
“One important message conveyed by our propositions is that even though algorithmic decision-making is a clear source of performance improvement through the automation of decisions, it should not be concluded that humans are out of the loop. Humans decide when decisions should (and should not) be made by algorithms. Humans specify, design and build algorithmic systems. Humans monitor and support their usage of fine-tuning parameters,” the authors write.
They end with one caveat about their guidance for implementing and using algorithms in organisational decision-making: “AI-supported decision-making is a fast-evolving field driven by the rapid growth of new technologies. A lot of the factors that we have looked at will quickly evolve in the coming years.”
AUTHORS
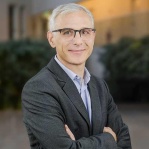
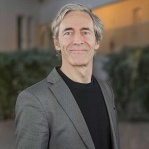
Campuses